Analyze the literature with text mining! Examples of checking the literature of childhood overweight and obesity
Abstract
The article presents text mining tools for processing the vast amount of literature. It is noted that significant developments have taken place in recent years and that several tools and methods are available for direct use by researchers. The authors present 7 methods in the field of childhood overweight and obesity, using 2 tools (Voyant Tools and VOSViewer), progressing from the simplest to the more complex analysis: (1) the design of the corpus, (2) trending of publication numbers, (3) the analysis of the frequency of terms, (4) collocation, (5) correlation, (6) context analysis, (7) concept network analysis, concept mapping - clustering. The use of methods and tools is illustrated with examples. These methods, used together can identify the most important developments and trends in the field, e.g. changes in interventions over the last ten years under study. Medicalization was also demonstrated during the study period. Crucially, the simpler text mining tools can be run on our usual IT tools and do not require any special expertise. However, additional sophisticated techniques mentioned in the discussion, in particular the use of artificial intelligence algorithms, require specific expertise and a computing toolkit of sufficient capacity. This is still difficult to access for public health researchers. In conclusion, the use of advanced text mining tools provides the opportunity for a rapid, quantitative, and transparent review of the literature, which becomes necessary when the number of publications in a given field exceeds the thousands to tens of thousands in the period under review.
References
Bartholomew, M. (2002) James Lind’s Treatise of the Scurvy (1753). Postgraduate Medical Journal, 78, 695–696. http://dx.doi.org/10.1136/pmj.78.925.695
Sur, R. L., & Dahm, P. (2011). History of evidence-based medicine. Indian journal of urology : IJU : journal of the Urological Society of India, 27(4), 487–489. https://doi.org/10.4103/0970-1591.91438
Balkányi, L., Lukács, L., & Cornet, R. (2021). Investigating the Scientific 'Infodemic' Phenomenon Related to the COVID-19 Pandemic. Yearbook of medical informatics, 30(1), 245–256. https://doi.org/10.1055/s-0041-1726483
Hendrigan, H. (2019). Mixing Digital Humanities and Applied Science Librarianship: Using Voyant Tools to Reveal Word Patterns in Faculty Research. Issues in Science and Technology Librarianship, (91). https://doi.org/10.29173/istl3
van Eck, N., J. & Waltman L. (2022) VOSviewer Manual. Manual for VOSviewer version 1.6.18. Leiden Universitate, CWTS Meaningful Metrics. https://www.vosviewer.com/documentation/Manual_VOSviewer_1.6.18.pdf
O'Mara-Eves, A., Thomas, J., McNaught, J., Miwa, M., & Ananiadou, S. (2015). Using text mining for study identification in systematic reviews: a systematic review of current approaches. Systematic reviews, 4(1), 5. https://doi.org/10.1186/2046-4053-4-5
Tao, D., Yang, P., & Feng, H. (2020). Utilization of text mining as a big data analysis tool for food science and nutrition. Comprehensive reviews in food science and food safety, 19(2), 875–894. https://doi.org/10.1111/1541-4337.12540
Chen, X., Cheng, G., Wang, F. L., Tao, X., Xie, H., & Xu, L. (2022). Machine and cognitive intelligence for human health: systematic review. Brain informatics, 9(1), 5. https://doi.org/10.1186/s40708-022-00153-9
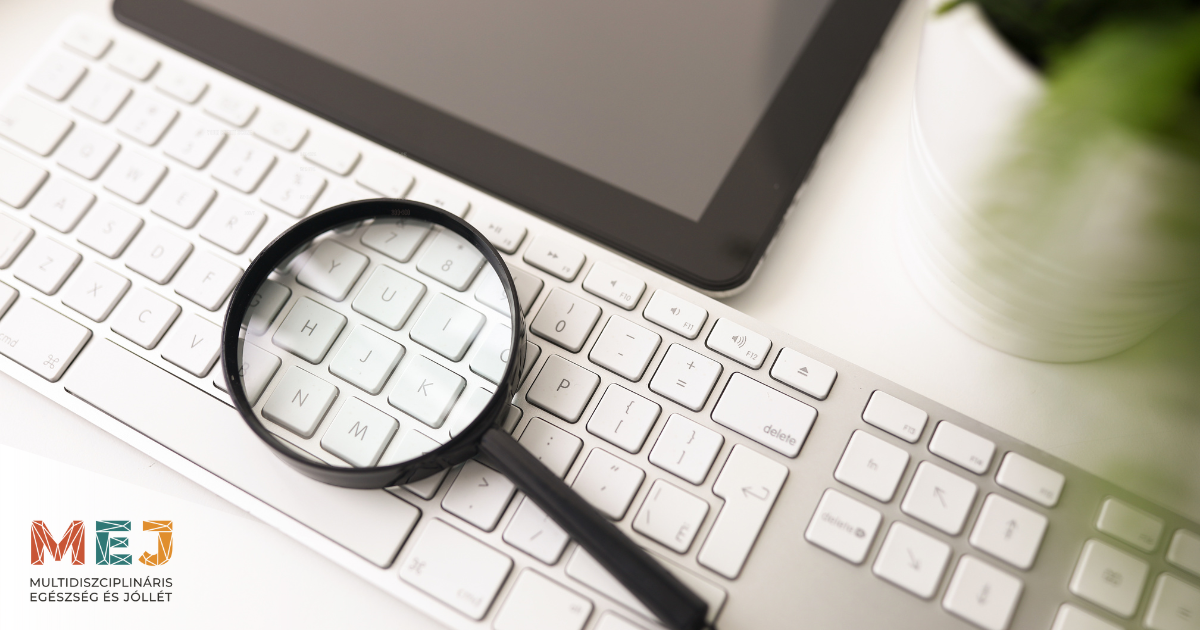