Idősor szinkron validitás elemzés kulcsszókutatáson alapuló neurális háló által előrejelzett olajárfolyam változáson
Absztrakt
Az olaj az egyik legfontosabb nyersanyag a világgazdaságban. Szinte minden szegmensben használják, logisztika, termelés, szolgáltatások eljuttatása vagy szolgáltató eljutása a szolgáltatási helyre stb. Egyszóval a teljes gazdaságot behálózza, ezért nagyon érzékenyen reagál a piac minden volatilitásra, áremelkedésre. A nagy felhasználók esetében egyértelmű a fontosság, de a lakossági szegmensben is nagy befolyással bír, mivel a fogyasztói kosár elemeit érinti, valamint a munkábajárási költséget változtatja stb. Így az aktorok számára fontos, hogy készülni tudjanak a változásra, amennyiben nagyobb kilengés van, úgy a gazdasági döntéseiket ennek fényében tudják meghozni, így elkerülve a kiszolgáltatottságot, valamint minimalizálni a volatilitásból eredő veszteségeket. Az olajforgalmazók, pontosabban az előállítók oligopol piacon működnek, ahol több esetben egyeztetik döntéseiket, változtatnak kitermelési mennyiségein, ami fokozza az előrejelzés bizonytalanságát. Vizsgálatunk folyamán vezető gazdasági folyóiratok cikkeit elemezzük kulcsszókutatással, illetve több egyéb változót alkalmazunk a Neurális Hálóval történő előrejelzés során, majd az így kapott idősor együttmozgását hasonlítjuk össze a valós olajár volatilitásával, ezzel felbecsülve, hogy mennyire előrejelezhetők a változások nem bizalmi információval rendelkező szereplők számára. Az idősor elemzést több módszerrel folytatjuk le, ezzel elkerülve a részrehajlást, illetve következtetve arra, hogy milyen módon alkalmas ez a rendszer a valós és nem feltétlenül várható piaci mozgások időben való előrejelzésére, így a gazdasági döntéseink időzítésére, hogy minél kisebb legyen az ebből fakadó negatív hatás.
Hivatkozások
Alaminos, D., Esteban, I., Salas, M.B. & Callejón, A.M., 2020. Quantum Neural Networks for Forecasting Inflation Dynamics, Journal of Scientific & Industrial Research. (79) 103-106. https://doi.org/10.56042/jsir.v79i2.68439
Baker, M.P. & Wurgler, J.A., 2007. Investor Sentiment in the Stock Market. SSRN Electronic Journal. https://doi.org/10.2139/ssrn.962706
Barberis, N., Shleifer, A. & Vishny, R., 1998. A model of investor sentiment. Journal of Financial Economy. (4) 307-343.
Dadgar Y., Keshavarz Gh. & Tyataraj A., 2006. The Analysis of Relationship Between Inflation and Economic Growth in Iran, Journal of Economic Literature (5) 59-88.
Daniel, K. & Titman, S. (1996). Evidence on the Characteristics of Cross Sectional Variation in Stock Returns. The Journal of Finance, 52(1), 1-33. https://doi.org/10.2307/2329554
DeLong, J.B., Shleifer, A., Summers, L.H. & Waldmann, R.J., 1990. Noise trader risk in financial markets. Journal of Political Economy. (98), 703-738.
Fama, E.F. & French, K.R., 1993. Common risk factors in the returns on stocks and bonds. Journal of Financial Economics, 33(1), 3-56. https://doi.org/10.1016/0304-405x(93)90023-5
Fama, E.F. & French, K.R., 1996. Multifactor Explanations of Asset Pricing Anomalies. The Journal of Finance. 51(1), 55-84. https://doi.org/10.1111/j.1540-6261.1996.tb05202.x
Fama, E.F., French, K.R., 1997. Industry cost of equity. Journal of Financial Economy. (43), 153–193.
Fan, Y., Liang, Q. & Wei, Y.M., 2008. A generalized pattern matching approach for multi-step prediction of crude oil price. Energy Economics, 30(3), 889-904. https://doi.org/10.1016/j.eneco.2006.10.012
Galeshchuk, S. & Demazeau, Y., 2017. Forecasting hungarian forint exchange rate with convolutional neural networks. International Conference on Behavioral, Economic, Socio-Cultural Computing (BESC). https://doi.org/10.1109/besc.2017.8256358
Gupta, R., & Kabundi, A. (2011). A large factor model for forecasting macroeconomic variables in South Africa. International Journal of Forecasting, 27(4), 1076-1088. https://doi.org/10.1016/j.ijforecast.2010.10.001
Heidari H. & Parvin S., 2009. Modeling and Forecasting Iranian Inflation with Time Varying BVAR Models, Iranian Journal of Economic Research (36) 59-84.
Jammazi, R. & Aloui, C., 2012. Crude oil price forecasting: Experimental evidence from wavelet decomposition and neural network modeling. Energy Economics, 34(3), 828-841. https://doi.org/10.1016/j.eneco.2011.07.018
Kamstra, M.J., Kramer, L.A. & Levi, M.D., 2000. Winter Blues: A SAD Stock Market Cycle. SSRN Electronic Journal https://doi.org/10.2139/ssrn.208622
Kumar, M., Moorthy, U. & Perraudin, W., 2003. Predicting emerging market currency crashes. Journal of Empirical Finance, 10(4), 427-454. https://doi.org/10.1016/s0927-5398(02)00068-3
Langcake, S. & Robinson, T., 2017. Forecasting the Australian economy with DSGE and BVAR models. Applied Economics, 50(3), 251-267. https://doi.org/10.1080/00036846.2017.1319558
Madhou, A., Sewak, T., Moosa, I. & Ramiah, V., 2019. Forecasting the GDP of a small open developing economy: an application of FAVAR models. Applied Economics, 1–12. https://doi.org/10.1080/00036846.2019.1679346
Mahdiani, M.R. & Khamehchi, E., 2016. A modified neural network model for predicting the crude oil price. Intellectual Economics, 10(2), 71–77. https://doi.org/10.1016/j.intele.2017.02.001
Moshiri, S. & Cameron, N., 2000a. Neural network versus econometric models in forecasting inflation. Journal of Forecasting, 19(3), 201-217. https://doi.org/10.1002/(sici)1099-131x(200004)19:3<201::aid-for753>3.0.co;2-4
Naderi, M., Khamehchi, E. & Karimi, B., 2018. Novel statistical forecasting models for crude oil price, gas price, and interest rate based on meta-heuristic bat algorithm, Journal of Petroleum Science and Engineering, https://doi.org/10.1016/j.petrol.2018.09.031
Neal, R. & Wheatley, S.M., 1998. Do Measures of Investor Sentiment Predict Returns? The Journal of Financial and Quantitative Analysis, 33(4), 523. https://doi.org/10.2307/2331130
Nordhaus, W.D., 2011. The Economics of Tail Events with an Application to Climate Change. Review of Environmental Economics and Policy, 5(2), 240-257. https://doi.org/10.1093/reep/rer004
Onimode, B., Alhassan, J., and Adepoju, S. (2015). Comparative study of inflation ratesforecasting using feed-forward artificial neural networks and auto-regressive (ar) models. International Journal of Computer Science Issues, 12.
statista.com, Distribution of oil production worldwide in 2021, by region [online] https://www.statista.com/statistics/277621/distribution-of-global-oil-production-by-region/ (2023.05.10.)
Subecz, Z., 2019. Event Detection and Classification in Natural Texts, Gradus 6(1), 16-21.
Swaminathan, B., 1996. Time-Varying Expected Small Firm Returns and Closed-End Fund Discounts. Review of Financial Studies, 9(3), 845-887. https://doi.org/10.1093/rfs/9.3.845
Thakur, G.S.M., Bhattacharyya, R. & Mondal, S.S., 2016. Artificial Neural Network Based Model for Forecasting of Inflation in India. Fuzzy Information and Engineering. 8(1), 87-100. https://doi.org/10.1016/j.fiae.2016.03.005
Tkacz, G., 2001. Neural network forecasting of Canadian GDP growth. International Journal of Forecasting, 17(1), 57–69. https://doi.org/10.1016/s0169-2070(00)00063-7
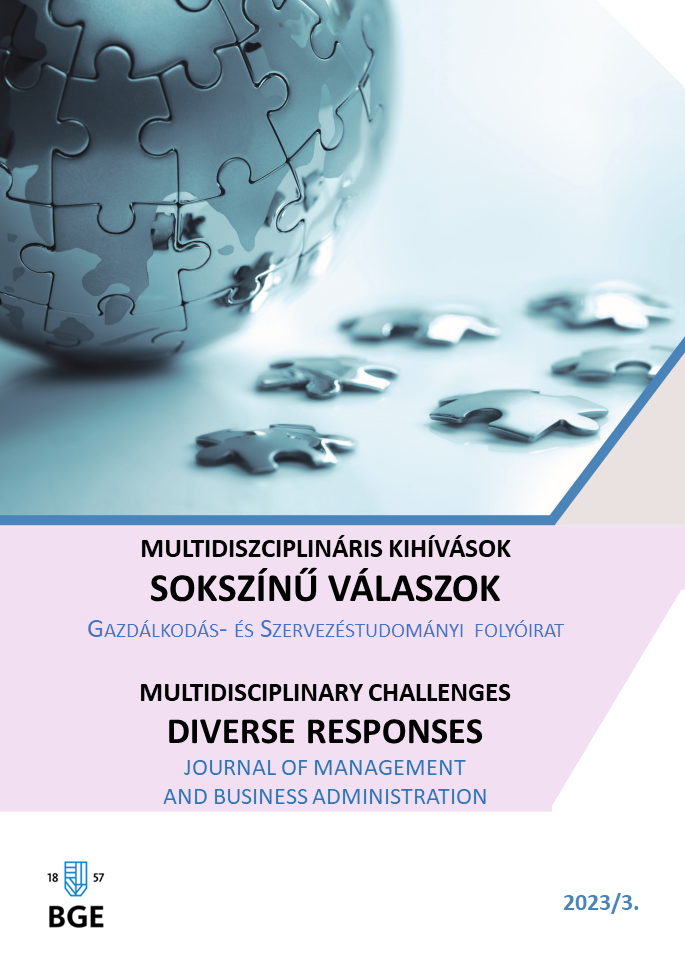